Data Silos vs Data Lakes: Which Strategy Drives Data Management?
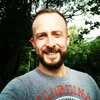
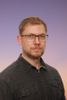
Updated May 15, 2023.
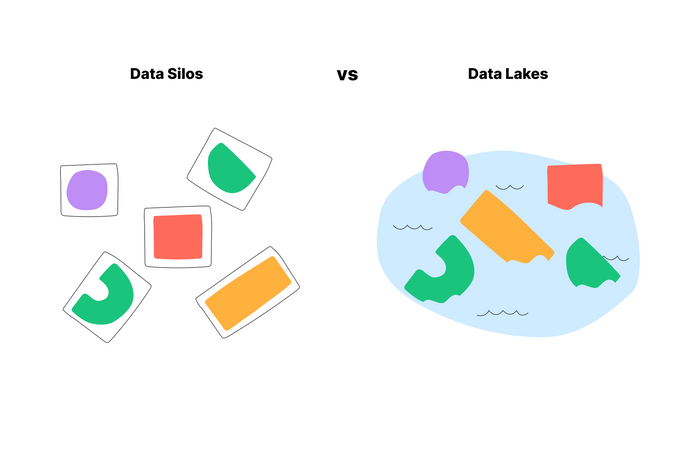
In today's digital era, businesses are inundated with data, making effective data management essential for making sense of this deluge of information. Data management solutions can provide businesses with the answers they need, or at least a starting point, to determine how to handle all this data. Data silos and data lakes are two different approaches to managing and storing large volumes of data or big data.
Here is a comprehensive outline of both concepts and how they drive data management.
What is a Data Silo?
Data silos in big data refer to isolated data repositories created within an organization as different departments or teams generate and store their own data.
This type of data management makes it challenging for businesses to search, access, and analyze data across departments, hindering effective collaboration and reducing process efficiency. They can also affect data integrity, as the same information may be stored in different databases, leading to inconsistencies and data synchronization issues.
As such, breaking down data silos is crucial for unlocking effective and unified data management.
Breaking Down Data Silos
There are several ways to break down data silos and enhance information sharing. These include:
- Changing Company Culture: Data silos may result from organizational structures that encourage departments to store data locally or keep information within their confines. Organizations need to communicate with employees and teams about the problems associated with data silos and the benefits of information sharing for better data management and analysis.
- Centralizing Company Data: Organizations need to pool all company data into a cloud-based data lake where it will be consolidated and easily accessed. This will provide a centralized location for all data and eliminate the need for departmental databases.
- Fostering Data Integration: Data integration involves combining data from different sources into a single unified view, providing a consistent and consolidated view of data. ETL (Extract, Transform, Load) is a popular approach to data integration, involving extracting data from source systems, transforming it to conform to the target system's format, and loading it into the target system.
- Creating a Data Governance Framework: Establishing a data governance framework that centralizes data access and control can prevent the creation of data silos. The framework should include policies and procedures for managing data quality, security, privacy, and compliance with using and accessing data.
This way, organizations can break down data silos, enhance information sharing, and promote collaboration across departments.
What is a Data Lake?
A data lake is a centralized repository that stores, processes, and secures data in various formats, including structured, semi-structured, and unstructured data. It facilitates the centralization of information, helping to break down data silos in organizations.
Data lakes offer an intelligent approach to data management, enabling cost-effective storage of large amounts of raw data in its native format, making it ready for AI/ML analytics.
How to Build a Data Lake?
Here is a broad outline of how to build a data lake:
- Identify Your Organization’s Data Goal: Before you start building a data lake, identify the data analytics goals of your organization. This will help you to determine the relevant data you need to store in the data lake.
- Set Up Cloud Storage: Data lakes are typically cloud-based and designed to support big data processing and analytics. Solutions like Amazon S3, Microsoft Azure, or Google Cloud offer scalability and cost-effectiveness.
- Prepare the Data: Ensuring data quality, consistency, and accuracy before loading it into the data lake is essential. Raw data can be messy and unstructured, making searching, analyzing, and deriving insights difficult. Data preparation involves data profiling, data cataloging, data backup, and archives.
- Enforce Security Policies: Data lakes can contain sensitive information. Therefore, it's important to implement access controls, encryption, and auditing to keep sensitive data safe and track access to the data.
- Make Data Available For Analytics: Analyzing and learning from the data can provide insights into business operations and improve decision-making.
✶ Find out how federated search integrates with data lakes
Overcoming Data Management Challenges
While breaking down data silos and embracing data lakes can help organizations become data-driven, it is easier said than done. Challenges include the learning curve associated with data lakes and their susceptibility to malware pollution. When malware "seeps" into data lakes, it can turn them into gold mines for malicious actors.
However, enabling uninhibited search capabilities can serve as an alternative to breaking down data silos, as it allows users to search through and across data repositories within an organization.
To this end, tools like Unleash offer a simple and easy-to-use solution that enables users to search every folder and application. This streamlines productivity and allows employees to quickly access the information they need.